Financial Forecasting: How to Predict Business Growth is crucial for any business aiming for sustainable success. Accurately predicting future performance allows for proactive strategic planning, informed resource allocation, and ultimately, increased profitability. This guide delves into the essential methods and models, equipping you with the knowledge to navigate the complexities of financial forecasting and make data-driven decisions that propel your business forward.
From understanding key growth drivers and collecting relevant data to employing various forecasting models and interpreting key financial metrics, we’ll explore a comprehensive approach. We’ll also cover crucial aspects like scenario planning, risk assessment, and effectively communicating your forecasts to stakeholders. By the end, you’ll possess a robust understanding of how to translate raw data into actionable insights for future growth.
Understanding Business Growth Drivers: Financial Forecasting: How To Predict Business Growth
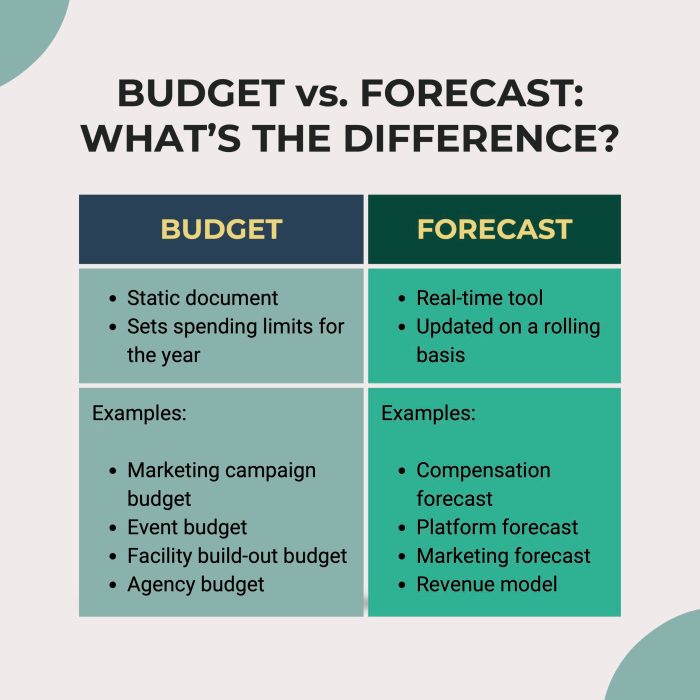
Accurate financial forecasting hinges on a thorough understanding of the factors driving business growth. Predicting future performance requires analyzing both internal capabilities and external market forces, recognizing that their interplay significantly shapes a company’s trajectory. Ignoring either aspect leads to inaccurate predictions and flawed strategic planning.
Internal and external factors intertwine to influence a company’s growth. Internal factors include operational efficiency, employee skill sets, innovative product development, and effective marketing strategies. External factors encompass market size, competition, economic conditions, regulatory changes, and technological advancements. For instance, a company with a highly efficient production process (internal) might struggle if the overall market demand declines (external). Understanding this interplay is crucial for realistic growth projections.
Get the entire information you require about Double Entry Accounting Explained with Examples on this page.
Market Trends and Projected Growth
Market trends significantly impact projected growth. Analyzing market size, growth rate, and customer behavior provides valuable insights into potential revenue streams. For example, the increasing demand for sustainable products presents a growth opportunity for companies in the eco-friendly sector. Conversely, declining demand for a particular product necessitates adjustments to forecasts and possibly a shift in business strategy. Analyzing historical data, conducting market research, and monitoring competitor activities allows for a more accurate prediction of market trends and their influence on future growth. Consider the example of the smartphone market: the initial explosive growth has slowed, requiring manufacturers to focus on niche markets and technological innovation to maintain their growth trajectory.
Innovation and Technological Advancements
Innovation and technological advancements are powerful drivers of business growth. Companies that consistently invest in research and development, and adapt to technological changes, are better positioned for long-term success. For example, the rise of e-commerce significantly altered the retail landscape, forcing traditional brick-and-mortar stores to adapt or risk obsolescence. Similarly, advancements in artificial intelligence and machine learning are transforming various industries, presenting both opportunities and challenges for businesses. Financial forecasts should account for the potential impact of disruptive technologies and the company’s ability to leverage them effectively. Companies that fail to adapt risk becoming irrelevant, impacting their growth potential dramatically.
Growth Strategies and Their Impact on Financial Forecasts
Different growth strategies lead to varying impacts on financial forecasts. Market penetration, focusing on increasing market share with existing products, generally leads to more predictable and incremental growth. Market development, expanding into new markets with existing products, involves higher risk but potentially higher rewards, leading to more variable growth projections. Product development, introducing new products to existing markets, often requires significant upfront investment but can yield substantial returns over time. Diversification, entering new markets with new products, carries the highest risk but offers the potential for significant growth if successful. Each strategy requires a distinct forecasting approach reflecting its inherent risks and potential returns. For example, a company adopting a diversification strategy might include contingency plans and more conservative growth projections in its financial forecasts to account for the inherent uncertainties involved.
Data Collection and Preparation for Forecasting
Accurate financial forecasting hinges on the quality and completeness of the data used. Gathering and preparing this data is a crucial first step, requiring meticulous attention to detail to ensure the reliability of your predictions. Without properly prepared data, even the most sophisticated forecasting models will yield inaccurate results.
Essential Data Points for Financial Forecasting
The specific data points required will vary depending on the nature of the business and the forecasting goals. However, some essential data points consistently contribute to accurate financial forecasting. These data points provide a comprehensive picture of the company’s financial health and performance trends.
These include:
- Revenue Data: Historical sales figures, broken down by product, region, and customer segment. This provides insight into sales trends and growth patterns.
- Cost Data: Detailed information on the cost of goods sold (COGS), operating expenses (rent, salaries, marketing), and other expenses. Analyzing cost structures helps identify areas for potential savings.
- Financial Statements: Income statements, balance sheets, and cash flow statements provide a comprehensive overview of the company’s financial performance over time. These statements are crucial for understanding profitability, liquidity, and solvency.
- Market Data: Industry trends, competitor analysis, and macroeconomic factors (interest rates, inflation) are essential for contextualizing the business’s performance and anticipating future market conditions. Ignoring external factors can lead to significant forecasting errors.
- Operational Data: Production capacity, inventory levels, customer acquisition costs, and other operational metrics provide valuable insights into the efficiency and effectiveness of the business operations.
Data Cleaning and Preparation Procedures
Before using data in forecasting models, it’s crucial to clean and prepare it to eliminate errors and inconsistencies. This step ensures the accuracy and reliability of your forecast. A systematic approach is vital to this process.
A step-by-step procedure includes:
- Data Consolidation: Gather data from various sources (spreadsheets, databases, etc.) and consolidate it into a single, consistent format. Inconsistencies in formatting can lead to errors in analysis.
- Data Validation: Check for data entry errors, inconsistencies, and outliers. This involves comparing data points against known values or expected ranges. For example, a negative revenue figure would immediately flag an error.
- Data Transformation: Convert data into a suitable format for analysis. This might involve converting dates, standardizing units, or creating new variables (e.g., calculating profit margins).
- Data Cleaning: Address inconsistencies and errors identified during validation. This may involve correcting errors, removing duplicates, or imputing missing values (discussed in the next section).
Handling Missing Data and Outliers
Missing data and outliers are common challenges in financial datasets. Addressing these issues appropriately is crucial for producing accurate forecasts. Different strategies can be employed depending on the nature and extent of the problem.
Methods for handling missing data include:
- Deletion: Removing rows or columns with missing data. This is only suitable if the amount of missing data is small and doesn’t significantly bias the results. This method is often less preferred as it can reduce the sample size.
- Imputation: Replacing missing values with estimated values. Common methods include using the mean, median, or mode of the available data. More sophisticated techniques, such as regression imputation, can also be employed. This approach preserves the sample size, but may introduce bias if not done carefully.
Outlier handling techniques include:
- Identification: Identify outliers using methods such as box plots or Z-scores. Outliers are data points significantly different from the rest of the data, often indicative of errors or unusual events.
- Removal: Remove outliers if they are deemed to be errors or if they significantly distort the analysis. This is done cautiously, as legitimate but extreme values may contain important information.
- Transformation: Transform the data (e.g., using logarithmic transformation) to reduce the influence of outliers. This approach retains the data, while reducing the disproportionate influence of extreme values.
Data Organization for Forecasting Models
Organizing data into a format suitable for forecasting models is crucial for efficient analysis. A well-structured dataset simplifies the modeling process and improves the accuracy of predictions. This typically involves creating a structured table.
An example of data organization for forecasting is shown below:
Year | Month | Revenue | Operating Expenses |
---|---|---|---|
2021 | January | 10000 | 5000 |
2021 | February | 12000 | 5500 |
2021 | March | 15000 | 6000 |
2022 | January | 11000 | 5200 |
2022 | February | 13000 | 5700 |
2022 | March | 16000 | 6200 |
Forecasting Methods and Models
Accurately predicting future business performance requires a robust understanding of various forecasting methods. The choice of method depends heavily on the nature of the data available, the desired level of accuracy, and the resources available. Broadly, forecasting methods are categorized as qualitative and quantitative.
Qualitative and Quantitative Forecasting Methods
Qualitative forecasting relies on expert judgment, intuition, and subjective opinions. This approach is useful when historical data is scarce or unreliable, or when dealing with emerging markets or new products where past trends offer little guidance. Quantitative forecasting, on the other hand, uses mathematical models and historical data to predict future outcomes. This approach is data-driven and aims for objectivity and precision. The choice between these approaches often involves a trade-off between the availability of data and the need for incorporating expert knowledge.
Examples of Quantitative Forecasting Models
Several quantitative models exist, each with its own strengths and weaknesses. These models range from simple to complex, reflecting the varying complexity of business environments and data sets.
Time Series Analysis
Time series analysis uses past data points over time to predict future values. This approach assumes that historical patterns will continue into the future. Simple methods include moving averages, where the forecast is the average of the previous ‘n’ periods. More sophisticated techniques include exponential smoothing, which assigns exponentially decreasing weights to older data points, giving more importance to recent observations. ARIMA (Autoregressive Integrated Moving Average) models are even more complex, capable of capturing more intricate patterns in time series data.
Advantages: Relatively simple to implement for basic models; readily available software support.
Disadvantages: Assumes future trends will mirror past trends; can be inaccurate if underlying patterns change; may not capture external factors.
Example: A retailer might use a moving average of past monthly sales to forecast sales for the next month. An exponential smoothing model might be more appropriate if sales exhibit seasonality or trend.
Regression Analysis, Financial Forecasting: How to Predict Business Growth
Regression analysis explores the relationship between a dependent variable (what we want to predict, e.g., sales) and one or more independent variables (factors influencing the dependent variable, e.g., advertising spend, price, economic indicators). Simple linear regression examines the relationship between one independent and one dependent variable. Multiple linear regression extends this to multiple independent variables. These models provide insights into the factors driving business growth and allow for more targeted interventions.
Advantages: Identifies key drivers of business growth; allows for scenario planning by changing independent variable values.
Disadvantages: Requires a strong understanding of the relationships between variables; can be sensitive to outliers; may require large datasets.
Example: A company might use multiple linear regression to forecast sales based on advertising expenditure, competitor pricing, and economic growth rates.
Accuracy and Applicability of Forecasting Models
The accuracy and applicability of different forecasting models vary greatly depending on the specific business scenario. Time series models are well-suited for situations with readily available historical data and relatively stable trends. Regression models are more appropriate when understanding the underlying drivers of business growth is crucial. In practice, a combination of methods is often used to improve forecasting accuracy and robustness. For instance, qualitative insights from market research might be integrated with quantitative forecasts from time series analysis to create a more comprehensive picture. The best model is the one that best fits the specific data and context, balancing complexity with accuracy and practicality.
Key Financial Metrics and Their Interpretation
Accurate interpretation of key financial metrics is crucial for effective financial forecasting. Understanding how these metrics interact and influence each other provides a clearer picture of a business’s health and potential for growth. This section will define several key metrics, explain their interpretation, and demonstrate their application in forecasting business growth.
Several key financial metrics provide valuable insights into a business’s performance and future prospects. These metrics, when analyzed together, paint a comprehensive picture, allowing for more accurate growth projections. Their effective use requires understanding not only their individual meanings but also their interrelationships.
Revenue Growth
Revenue growth represents the percentage change in a company’s revenue over a specific period. It’s a fundamental indicator of a company’s overall performance and market position. A consistently increasing revenue stream suggests strong market demand and effective business strategies. Conversely, declining revenue can signal underlying issues requiring immediate attention. In forecasting, revenue growth is often used as a baseline for projecting future income and related financial metrics. For example, if a company experienced 10% revenue growth last year, a conservative forecast might project 8-12% growth for the next year, taking into account market conditions and potential internal factors.
Profit Margin
Profit margin represents the percentage of revenue remaining after deducting all costs. Different types of profit margins exist, including gross profit margin (revenue less cost of goods sold), operating profit margin (revenue less cost of goods sold and operating expenses), and net profit margin (revenue less all costs, including taxes and interest). These margins indicate the efficiency and profitability of a company’s operations. Higher profit margins suggest better cost control and pricing strategies. In forecasting, profit margins are crucial for projecting profitability and assessing the impact of changes in revenue, costs, or pricing. For instance, if a company anticipates increased material costs, it might need to adjust its pricing strategy to maintain its desired profit margin, influencing its revenue growth projection.
Return on Investment (ROI)
Return on Investment (ROI) measures the profitability of an investment relative to its cost. It’s expressed as a percentage and calculated as (Net Profit / Cost of Investment) * 100. ROI is a valuable metric for evaluating the effectiveness of various business initiatives, such as marketing campaigns or new product launches. In forecasting, ROI helps assess the potential return from different investment strategies and inform resource allocation decisions. For example, a company might forecast the ROI of a new marketing campaign to determine if it’s worth the investment based on projected revenue increases and campaign costs.
Key Financial Metrics Table
Metric | Meaning and Interpretation |
---|---|
Revenue Growth | Percentage change in revenue over time; indicates market demand and business effectiveness. High growth suggests strong performance; low or negative growth indicates potential problems. |
Profit Margin (Gross, Operating, Net) | Percentage of revenue remaining after deducting various costs; reflects operational efficiency and profitability. Higher margins indicate better cost control and pricing strategies. |
Return on Investment (ROI) | Measures the profitability of an investment relative to its cost; helps evaluate the effectiveness of various business initiatives. High ROI suggests a successful investment. |
Impact of Metric Changes on Growth Projections
Changes in these key financial metrics directly influence future growth projections. For example, a decline in profit margins due to increased operating costs might necessitate adjustments to pricing strategies or cost-cutting measures, impacting projected revenue growth. Conversely, a significant increase in ROI from a new product launch could lead to increased investment in that product line, driving higher revenue and overall growth. Analyzing trends and potential changes in these metrics is crucial for developing realistic and accurate growth forecasts. A company experiencing consistent revenue growth but declining profit margins might need to investigate cost structures or pricing strategies to ensure long-term sustainability and profitability, potentially altering its future growth projection downward.
Scenario Planning and Risk Assessment
Financial forecasting, while aiming for accuracy, inherently deals with uncertainty. To improve the robustness and reliability of your forecasts, incorporating scenario planning and a thorough risk assessment is crucial. This process allows you to anticipate potential disruptions and adjust your strategies proactively, leading to more resilient financial planning.
Scenario planning helps to visualize different potential futures and their impact on your business’s financial performance. By considering a range of possibilities, from optimistic to pessimistic, you can develop more adaptable and resilient plans. This proactive approach allows for better resource allocation and decision-making in the face of unexpected events.
Developing Multiple Growth Scenarios
A robust framework for scenario planning involves creating three distinct scenarios: a best-case, a worst-case, and a most-likely scenario. The best-case scenario Artikels the most optimistic outcome, based on favorable market conditions and successful execution of strategies. The worst-case scenario explores the least favorable conditions and potential setbacks. Finally, the most-likely scenario represents a realistic projection, balancing optimistic and pessimistic factors. Each scenario should include detailed assumptions about key drivers of growth, such as market share, pricing, and operating expenses. For example, a best-case scenario for a tech startup might involve exceeding user acquisition targets, securing significant funding, and launching a successful product with minimal competition. Conversely, the worst-case scenario might involve lower-than-expected user engagement, delays in securing funding, and facing fierce competition from established players. The most-likely scenario would then represent a balanced view of these possibilities.
Identifying Potential Risks and Uncertainties
Several factors can significantly impact the accuracy of financial forecasts. These risks and uncertainties can be categorized into internal and external factors. Internal risks might include operational inefficiencies, supply chain disruptions, or inadequate management. External risks include macroeconomic fluctuations (e.g., inflation, recession), changes in government regulations, or shifts in consumer preferences. A comprehensive risk assessment involves identifying all potential risks, analyzing their likelihood of occurrence, and evaluating their potential impact on the financial forecast. For instance, a sudden increase in raw material prices (external risk) could significantly impact a manufacturing company’s profitability, while a failure to launch a new product on time (internal risk) could affect revenue projections.
Incorporating Risk into Financial Forecasting Models
Several methods can incorporate risk into your forecasting models. Sensitivity analysis examines how changes in key assumptions (e.g., sales growth, cost of goods sold) affect the forecast. Monte Carlo simulation uses probability distributions for uncertain variables to generate a range of possible outcomes, providing a more comprehensive view of risk. For instance, instead of using a single sales growth rate, a Monte Carlo simulation might use a range of growth rates with associated probabilities, generating a distribution of possible revenue outcomes. This allows for a better understanding of the uncertainty surrounding the forecast.
Strategies for Mitigating the Impact of Identified Risks
Once risks are identified and assessed, strategies should be developed to mitigate their potential impact. These strategies could involve diversifying revenue streams, hedging against price fluctuations, improving operational efficiency, or building contingency plans. For example, a company facing potential supply chain disruptions might diversify its sourcing to multiple suppliers, reducing its reliance on any single vendor. Similarly, a company concerned about inflation could implement pricing strategies to offset rising costs. Proactive risk management significantly enhances the resilience of the financial forecast and reduces the likelihood of significant negative surprises.
Visualizing and Communicating Forecasts
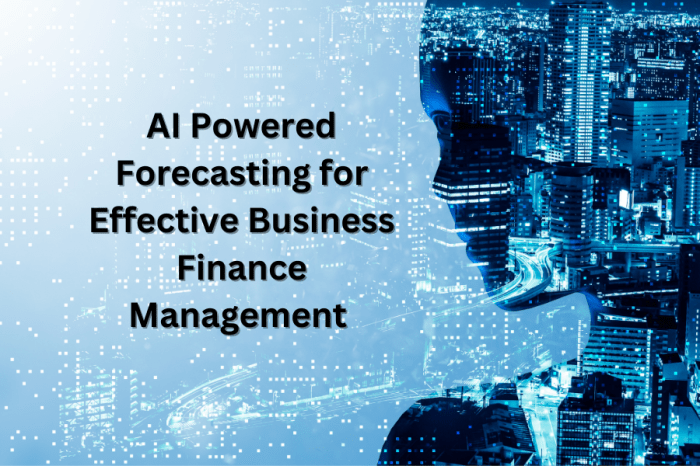
Effective visualization and clear communication are crucial for translating complex financial forecasts into actionable insights for stakeholders. A well-presented forecast not only clarifies predicted outcomes but also fosters trust and facilitates informed decision-making. This section details strategies for creating compelling visuals, structuring a concise report, and effectively communicating the forecast, along with addressing potential concerns.
Compelling Visual Representations of Forecasts
Visualizing financial forecasts using appropriate charts and graphs significantly improves understanding and impact. For instance, a line chart effectively displays projected revenue growth over time, clearly showing trends and potential inflection points. Imagine a line chart with “Revenue (in millions)” on the Y-axis and “Year” on the X-axis, plotting projected revenue for the next five years. A clear upward trend would immediately communicate positive growth expectations. To add context, shaded areas could represent confidence intervals, highlighting the range of possible outcomes based on the forecast’s inherent uncertainty. Similarly, a bar chart could compare projected revenue across different product lines or geographic regions, allowing for quick identification of high-performing areas and potential areas for improvement. For example, bars representing each product line’s projected revenue for the next year could be color-coded to enhance visual appeal and clarity. Finally, a combination chart could overlay projected revenue with projected expenses, allowing for a visual representation of projected profitability.
Organizing Forecast Results into a Clear and Concise Report
The forecast report should be structured logically, prioritizing clarity and conciseness. Begin with an executive summary providing a high-level overview of the key findings and recommendations. This should be followed by a detailed section outlining the methodology used, including data sources and assumptions made. Subsequent sections should present the forecast results, using visuals as described above to support the narrative. Clearly label all charts and graphs with appropriate titles and axis labels. Finally, include a section addressing potential risks and uncertainties associated with the forecast. The report should conclude with a summary of key implications and recommended actions. An example could be a report structured with sections like Executive Summary, Methodology, Revenue Projections, Expense Projections, Profitability Analysis, Risk Assessment, and Recommendations.
Effective Strategies for Communicating the Forecast to Stakeholders
Effective communication requires tailoring the message to the audience’s level of understanding and their specific interests. For executive-level stakeholders, a concise presentation focusing on key performance indicators (KPIs) and strategic implications is most effective. For operational teams, a more detailed explanation of the underlying assumptions and methodologies might be necessary. Consider using interactive dashboards that allow stakeholders to explore the data dynamically, drilling down into specific areas of interest. Presentations should use clear and simple language, avoiding technical jargon where possible. Regular updates and open communication channels are crucial for maintaining transparency and addressing any concerns.
Addressing Questions and Concerns about the Forecast’s Assumptions and Limitations
Transparency regarding the forecast’s limitations is paramount. Proactively address potential questions and concerns by explicitly stating the assumptions made during the forecasting process. For instance, if the forecast assumes a specific economic growth rate, clearly state this assumption and explain the potential impact of deviations from this rate. Acknowledge the inherent uncertainties involved in forecasting and present sensitivity analyses showing the impact of changes in key assumptions. This demonstrates a realistic understanding of the limitations and enhances credibility. For example, a table showing projected revenue under different economic growth scenarios (optimistic, baseline, pessimistic) would highlight the range of potential outcomes and associated uncertainties.
Final Summary
Mastering financial forecasting empowers businesses to transition from reactive to proactive decision-making. By understanding the interplay between internal factors, market trends, and various forecasting techniques, you can build a strong foundation for future success. Remember that while models provide valuable guidance, continuous monitoring, adaptation, and a healthy dose of informed intuition are vital for navigating the dynamic business landscape. Armed with the knowledge gained here, you are well-equipped to confidently predict and shape your business’s growth trajectory.
Question & Answer Hub
What software is best for financial forecasting?
Many options exist, from spreadsheet software like Excel to dedicated financial planning and analysis (FP&A) software. The best choice depends on your business needs and budget. Consider factors like ease of use, scalability, and integration with existing systems.
How often should I update my financial forecast?
The frequency depends on your industry and business volatility. Monthly updates are common for many businesses, while some might opt for quarterly or even annual reviews. More frequent updates are generally recommended for businesses operating in rapidly changing markets.
What are the limitations of financial forecasting?
Forecasting inherently involves uncertainty. Unforeseen events (e.g., economic downturns, natural disasters) can significantly impact accuracy. It’s crucial to acknowledge these limitations and incorporate risk assessment into your models.